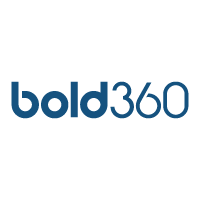
Bold360
View Brand PublisherHow a successful AI debut prompted PolicyBazaar to venture into a brand new business vertical with DocPrime
Ashish Gupta, CEO – DocPrime.com and CTO – PolicyBazaar.com shares how Artificial Intelligence is successfully transforming the business and customer experience for their new healthtech venture as well as India’s leading online insurance platform.
We run a large call centre with 8,000 people, and handle around 3-4 million policies a year. Our consumer base consists of about 12 million people.
When we started our AI journey one-and-a-half years ago, the whole idea was to explore whether we could aid a better customer experience by automating processes in real time. Also, we wanted to make it less challenging operationally both in terms of the number of people we have to manage, while bringing in more measurability. We started to explore how we could automate some of our processes.
Chat was one of the first areas that we decided to work on. In our motor insurance journey, we found that customers using the chat function were converting at double the of the non-chat customers. At the time, chat comprised only about 8-9 percent of our customer base but registered better conversions with more efficiency. So, we asked ourselves: how do we scale up?
Improving productivity, enhancing customer experience
That is when we came up with our first AI project -- a chat bot we built in-house backended by Google Dialogflow.
Over the next year, we expanded this initiative. We launched an assisted bot, which led the conversation, with a human taking over wherever it was not able to answer the customer. All such errors were recorded and we trained the system to improve it.
When it was an early stage product, it handled 20 percent of the responses and 80 percent was still handled by human beings. Over the next 6-8 months we were able to change the 20 percent number and bring it up to 75-80 percent.
A nice ‘side-effect’ is that out attrition rates have reduced dramatically by less than half.
It also helped us improve the quality and bring down the number of flags, which is the delay in response to a customer. Typically, we benchmark a flag at two minutes. This is considered bad customer experience. Earlier, our agents would be busy handling multiple customers. Now, these flags have come down from 35 percent to just 7-8 percent now.
From a business perspective, I am handling fewer people, which is operationally less challenging. Also, since fewer employees are leaving, there is a better legacy, and their training is retained.
In the motor insurance sector, almost 50 percent of our sales are via chat. Earlier, the chat volume was 8-9 per cent.
Launching new business vertical driven by success with AI
The success of our first AI journey encouraged us to take more steps on this front.
In fact, we are so confident about our chat processes that we have launched a new venture called DocPrime.com, an online healthcare platform, which connects patients with doctors in real time. This is a chat-first venture and is quite unique as not many have come up with a chat first interface especially in the medical space. We have doctors dedicated to us at the backend, and are building in an AI layer. Currently, we handle around 2,000 chats a day and are trying to scale it up further.
The next level we are working on is voice. In our call centre, we have 10 million minutes of talk time every month. This makes for very rich data that contains answers to customer preferences, nuances, likes and dislikes, the kind of queries we got, whether our agents were able to answer and did they pitch the right product. To ascertain quality of the call, we used to sample 1-2 per cent of the audio recordings, which did not give great insights.
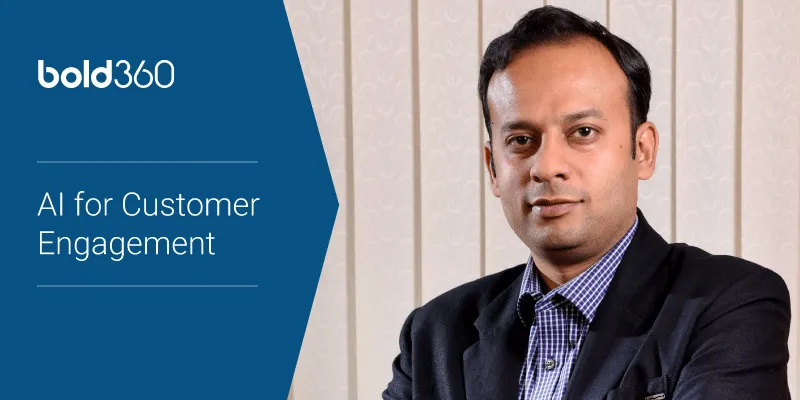
Instant alerts to improve quality consciousness
In the last one year, we have built a complete voice to text engine of our own focused on Hindi over telephony. Initially, we tried working with a third- party solution, but realised none gave the quality we needed. So we ended up building our own.
Now, every call is directly transcribed and saved in our database. Based on this we are able to perform various levels of analytics. We get automated alerts on triggers. These could be simple instances of what an agent should never do, such as asking for a mobile number or credit card information. Then we have triggers for advanced issues like pitching a maternity plan to a customer who is 45 years old. Or a customer called from a particular geography where two or three insurers are very strong and our agent pitched outside these.
We have a built-in mechanism and in case of any trigger an alert is sent out across the board, from the agent, the manager, to the head of sales. This helps us understand what went wrong and discuss it with the concerned people.
It has also helped us make significant co-relation between what the customer is saying, what sold, what did not sell and bring in improvements in our processes. We discovered that a lot of customers were talking about cashless networks in the voice recordings, and we immediately built cashless networks as part of our product journey itself.
Next step: introducing automation in video format
Voice is the next big thing. We have actually built an automated voice bot that we will launch later this month. Say, you bought motor insurance from us, we mailed you a soft copy, and for some reason you did not receive it -- it either went into your spam folder or got deleted by accident. You call us saying you haven’t received the soft copy. Earlier human agent would take your call, refer to your booking ID, download the PDF of the policy document and then mail it to you. None of this required human intelligence.
Our voice-to-text conversion engine does real time analysis on what the customer is asking for, and based on that respond to the customer right away. So if you ask for a soft copy, we can mail you a soft copy in real time, right within that call.
We are now building a voice-based chatbot for DocPrime.com. You have helplines for diseases like dengue fever, where people call to get their symptoms clarified. We want to build a voice bot that will respond to queries about the symptoms and suggest whether you might need to talk to a doctor or if the symptoms do not indicate a particular disease. We are trying to see how we can automate some aspects of a voice call. We have the tech part in place. Since the medical field is far more complicated in terms of regulations and risks, we are rigorously testing it. We are also trying to automate report validation where users can upload reports and our engine can help suggest further course of action.
Voice and chat are two things that have been most successful. Now we are starting to work on video. We have a video application in our PolicyBazaar app, where users can take a video of the damage on their car and upload it. Their claims are approved based on the video itself. This has proved to be very good since it has doubled our conversion rate. Right now, this process is manual. What we are trying to do is instead of somebody manually trying to look at these videos, we are building an AI layer, which can actually detect scratches and dents automatically.
In the next level, we want to automate the classification of complaints and problems. Initially, we want to automate 20 percent and will continue to work on introducing more automation till we reach 70-80 percent. We will hopefully release a version by the end of the year.
Challenge of building a quality product for a local ecosystem
Our challenge is being a local system. When we started off, we never wanted to build some of these engines. A lot of these voice to text engines were either built by Chinese tech companies or by US tech companies. These did not work out very well in the Indian landscape. One of our challenges has been adapting all of this technology to the Indian ecosystem, while making it cost-effective. That’s because we are transcribing every single call every day, so our performance has to be good. But it cannot be very expensive as we need to derive value from it.
So having to build it ourselves, and optimising it has been a challenge -- especially getting the content for training organised. But now we have been able to do it so I am quite happy about where we are right now.